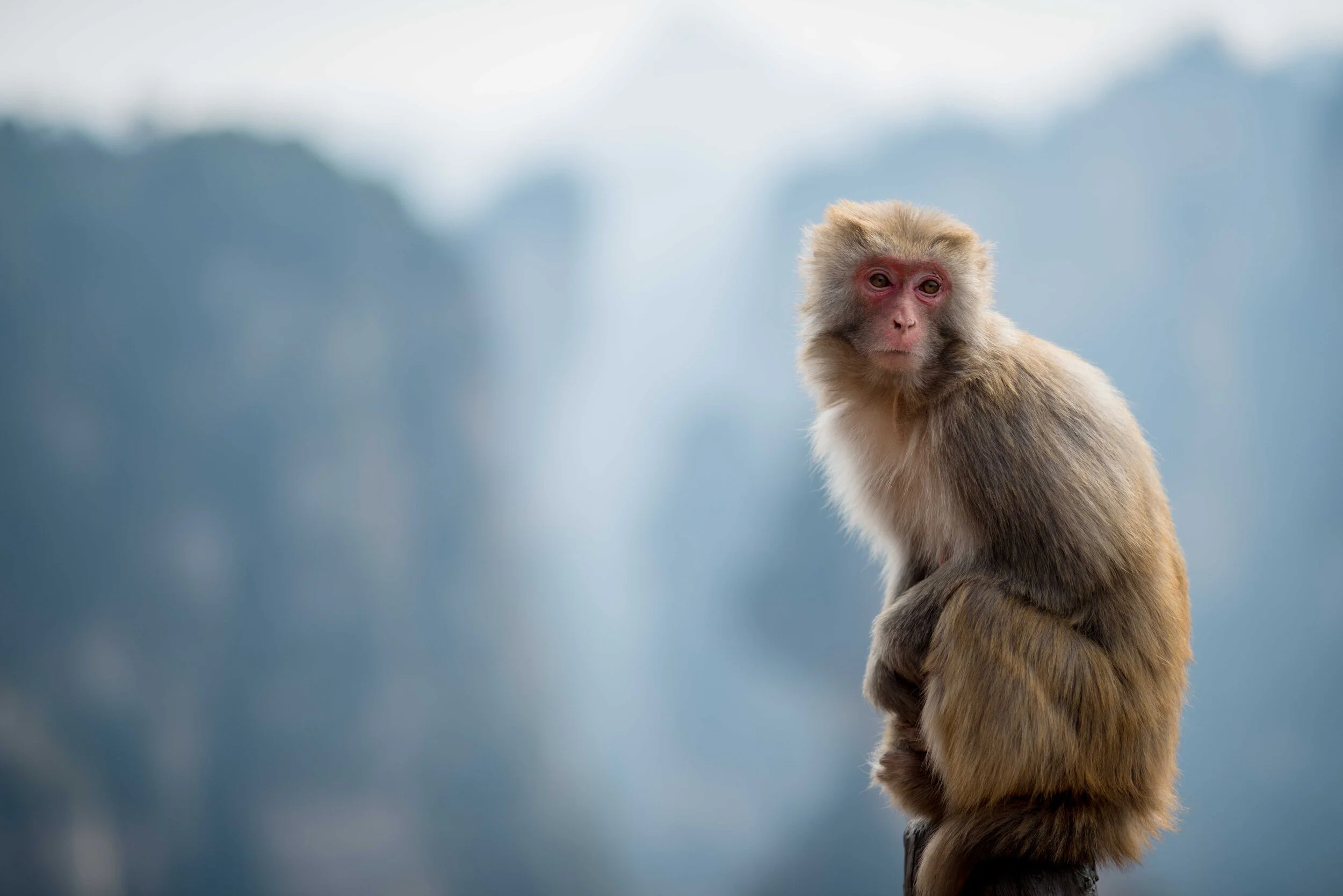
Scalable Electrode Technology for High Resolution Chronic Recording of Brain
Stuart F. Cogan, Department of Bioengineering
University of Texas, Dallas
Joseph J. Pancrazio, Department of Bioengineering
University of Texas, Dallas
People: Kevin Bodkin
Chronically implanted microelectrode arrays (MEAs) for recording extracellular neural activity are central to scientific studies of neural circuit function in behaving animals. These studies seek to understand how neurons encode information and how neural signals can be decoded to provide insights into brain learning and dysfunction. The majority of MEAs, especially those available commercially, are fabricated from silicon and leverage techniques associated with integrated circuit microfabrication and packaging. For MEAs, the major limitations for chronic neural recording are the reactive tissue response that encapsulates electrodes and kills or damages neurons in the vicinity of the electrode and the degradation and failure of materials used in MEA fabrication. An effective means of minimizing the foreign body response is the use of ultramicroelectrode MEAs (uMEAs) with subcellular cross-sectional dimensions. In related work, our colleagues, Drs. Stuart Cogan and Joe Pancrazio at the University of Texas (Dallas) have demonstrated that carbon-fiber uMEAs substantially evade a foreign body response and have been shown to provide stable chronic neural recordings in small-animal models. However, a scalable manufacturing process for carbon-fiber uMEAs has not emerged. This project is aimed at developing and demonstrating the chronic stability and reliability of uMEAs based on amorphous silicon carbide (a-SiC) and fabricated by industry-standard thin-film processes. Cogan and Pancrazio’s groups will develop a fabrication process for a-SiC uMEAs with 32 to 128 ultramicroelectrodes, which we will test in monkeys. We anticipate the proposed a-SiC uMEAs will impact the neuroscience community by providing a highly stable neural interface that allows single-unit and ensemble recording that is not possible with current multielectrode recording devices. We expect a-SiC uMEAs will provide new insights into the neural networks and changes in neural circuitry that may accompany behavior and adaption.