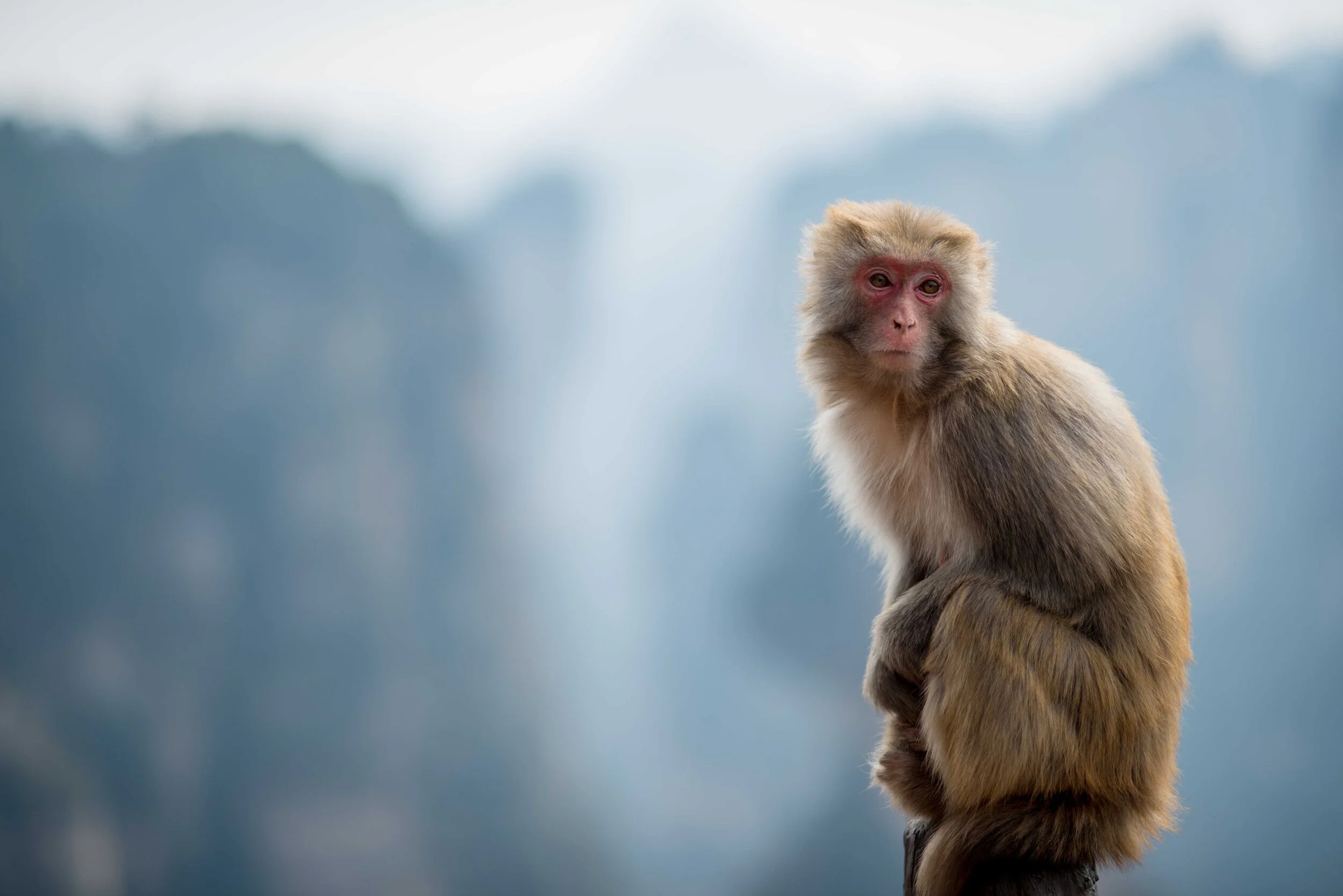
An Intracortical Brain-Computer Interface Model for High Efficiency Development of Closed-Loop Neural Decoding Algorithms
Zachary C. Danziger, Department of Biomedical Engineering
Florida International University
People: Eric Gasper, Aajan Quail
An intracortical brain-computer interface (iBCI) is used to record electrical signals directly from a person’s brain, predict their intention from those signals, then control an assistive device (e.g., a computer cursor, prosthetic limb, or powered wheelchair) according to those intentions. This technology enables severely paralyzed people to interact with the world. However, designing robust algorithms to extract intent from recordings of single neurons is extremely challenging, in large part because of the very limited access to humans, or even monkeys, from whom these invasive recordings can be made.
In this project, led by Dr. Zach Danziger at Florida International University, we will develop a model iBCI system that generates real-time biomimetic neural data by capturing the high-degree-of-freedom finger movements of able-bodied human subjects. To accomplish this, we will first construct a recurrent neural network (RNN) trained to predict the motor cortex activity of a monkey from the monkey’s finger kinematics. We will track finger kinematics using DeepLabCut, a markerless motion tracking system. Once the RNN is trained, its weights will be fixed and finger kinematics from a human subject will be used as the RNN inputs. This process will generate emulated neural activity, controlled in real time by the subject’s finger movements. The emulated neural activity can be passed to iBCI decoding algorithms that control computer cursors or other physical devices, allowing human subjects to control these devices through any given test decoder in real time, closed-loop conditions. Danzigers’ group will validate the emulated neural data by comparing subject performance, learning rates, and control strategies, to those of published experiments with invasive BMIs, including current state-of-the-art decoders. We will also use the iBCI model to evaluate novel decoder designs, and to determine the features of neural dynamics that are consistent across common iBCI tasks to help focus decoder development on those features.